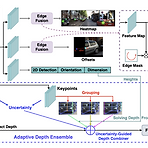
world center구하려면 3D object의 image에 투영된 center($u_{c}$, $v_{c}$)와 world depth($z$)가 필요하다. 이 논문에서는 크게 이 두 가지를 분리해서 구한다. 이 논문은 CenterNet의 확장이라고 볼 수 있다. CenterNet은 heatmap head, offset head, dimension head로 나누어져있다. heatmap head, offset head는 비슷한 기능을 하지만 3D point를 구하고, 이미지 안쪽과 이미지 바깥쪽에 있어 occlusion된 object를 구분하여 center를 구한다는 차이가 있다. Dimension head는 dimension orientation, 2d detection도 같이 구한다는 차이가 있다...
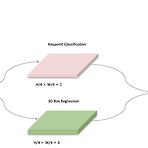
이 논문은 이미지에서 인식된 obeject(차량)의 실제 bounding box를 중심/크기/각도로 나누어 정보를 얻어 구할 수 있게 하는 모델을 제안하고 있다. 하나의 이미지에서 차량의 실제 정보를 얻을 수 있는(3D object detection) one-stage architecture를 제안하였으며, 중심/크기/각도로 분리하여 구하는 방식(multi-step disentanglement approach)을 제안하였다. Backbone - DLA(Deep Layer Aggregation)-34 수정해서 사용 - 모든 hierarchical aggregation을 DCN(Deformable Convolutional Networks)으로 대체 hierarchical aggregation은 같은 계층의 ..
- ML Pipeline
- TRACKING
- 디지털신호처리
- deeplearning
- design pattern
- OS
- image
- 운영체제
- Raspberry Pi
- controllable GAN
- Operating System
- DSP
- MLOps
- AI
- feature
- machine learning
- pcb
- Building Basic GAN
- depthmap
- depth
- Depth estimation
- conditional GAN
- mode collapse
- 딥러닝
- 3d object detection
- ML
- Deep learning
- Gan
- 신호처리
- Generative Model
- Total
- Today
- Yesterday
일 | 월 | 화 | 수 | 목 | 금 | 토 |
---|---|---|---|---|---|---|
1 | 2 | 3 | 4 | |||
5 | 6 | 7 | 8 | 9 | 10 | 11 |
12 | 13 | 14 | 15 | 16 | 17 | 18 |
19 | 20 | 21 | 22 | 23 | 24 | 25 |
26 | 27 | 28 | 29 | 30 | 31 |