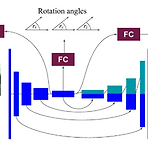
이 논문은 하나의 이미지의 depth, egomotion, object motion, and camera intrinsics을 동시에 학습하는 방식을 제안한다. motion-prediction network는 camera motion, motion of every pixel with respect to the background, and the camera intrinsics(focal lengths, offsets and distortion) 학습하고, 다른 network는 depth maps을 학습한다. 또한 occlusion 변화를 반영하기 위한 loss도 제안한다. 전체적인 Loss를 구하는 방식과 개념은 이전에 정리했던 논문에 기반하므로 이것을 참고하면 도움이 될 것이다. Learning the..
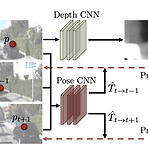
이 논문은 unsupervised한 방법으로 image depth를 구하는 모델을 학습시키는 방법이다. 학습에는 sequential한 이미지가 필요하고, 각 이미지의 pose를 이용해 한 이미지의 point들을 다른 이미지로 projection하고, projection된 위치의 점과 Intensity를 비교해 loss를 구한다. 여기서 pose도 model output이므로 gt없이 loss 계산이 가능해진다. Overview 조금 더 구체적으로 살펴보면 다음과 같은 단계로 나누어 생각해 볼 수 있다. 1. $t$에서의 이미지 $I_{t}$를 depth CNN에 통과시켜 Depthmap $\hat{D_{t}}(p)$를 구한다.(Depth map은 모든 이미지 픽셀에 대한 depth를 가지고 있다.) 2...
- TRACKING
- ML Pipeline
- feature
- deeplearning
- Operating System
- controllable GAN
- ML
- OS
- image
- depth
- machine learning
- design pattern
- Building Basic GAN
- Depth estimation
- Deep learning
- Gan
- AI
- conditional GAN
- 운영체제
- 신호처리
- pcb
- Raspberry Pi
- mode collapse
- 딥러닝
- Generative Model
- MLOps
- DSP
- 3d object detection
- depthmap
- 디지털신호처리
- Total
- Today
- Yesterday
일 | 월 | 화 | 수 | 목 | 금 | 토 |
---|---|---|---|---|---|---|
1 | ||||||
2 | 3 | 4 | 5 | 6 | 7 | 8 |
9 | 10 | 11 | 12 | 13 | 14 | 15 |
16 | 17 | 18 | 19 | 20 | 21 | 22 |
23 | 24 | 25 | 26 | 27 | 28 |