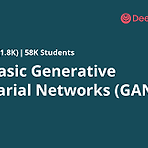
※ Coursera의 Build Basic Generative Adversarial Networks (GANs) 강의를 듣고 작성한 글입니다. Week4에서는 conditional GAN과 controllable GAN에 대해 학습합니다. Conditional & Unconditional GAN class가 있어서 class에 해당하는 이미지를 생성하는 것이 conditional GAN입니다. Unconditional이라면 어떤 class의 이미지가 생성될지 모르는 것이죠. Conditinoal gan은 one-hot vector를 사용하여 class에 대한 정보를 넣어줍니다. generator에는 1차원의 noise vector가 들어가므로 one-hot vector를 noise vector 뒤에 co..
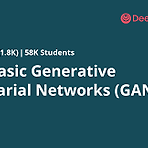
※ Coursera의 Build Basic Generative Adversarial Networks (GANs) 강의를 듣고 작성한 글입니다. Week 1에서는 GAN의 기본적인 구조에 대해 학습합니다. Generative Model 이란? 이름 그대로 무언가를 생성하는 모델을 generative model이라고 말합니다. 이를 discriminative model과 비교해볼게요. Discriminative model은 개와 고양이를 나누듯 class를 나누는 작업을 수행합니다. 어떤 이미지 feature가 input으로 들어가면 개인지, 고양이인지하는 class로 output이 나온게 됩니다. Generative model은 이미지를 생성해주는 모델입니다. input과 output이 generative..
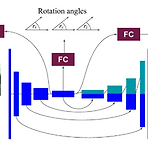
이 논문은 하나의 이미지의 depth, egomotion, object motion, and camera intrinsics을 동시에 학습하는 방식을 제안한다. motion-prediction network는 camera motion, motion of every pixel with respect to the background, and the camera intrinsics(focal lengths, offsets and distortion) 학습하고, 다른 network는 depth maps을 학습한다. 또한 occlusion 변화를 반영하기 위한 loss도 제안한다. 전체적인 Loss를 구하는 방식과 개념은 이전에 정리했던 논문에 기반하므로 이것을 참고하면 도움이 될 것이다. Learning the..
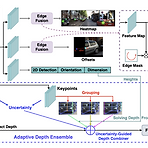
world center구하려면 3D object의 image에 투영된 center(uc, vc)와 world depth(z)가 필요하다. 이 논문에서는 크게 이 두 가지를 분리해서 구한다. 이 논문은 CenterNet의 확장이라고 볼 수 있다. CenterNet은 heatmap head, offset head, dimension head로 나누어져있다. heatmap head, offset head는 비슷한 기능을 하지만 3D point를 구하고, 이미지 안쪽과 이미지 바깥쪽에 있어 occlusion된 object를 구분하여 center를 구한다는 차이가 있다. Dimension head는 dimension orientation, 2d detection도 같이 구한다는 차이가 있다...
- Operating System
- ML Pipeline
- MLOps
- machine learning
- depthmap
- conditional GAN
- Raspberry Pi
- 신호처리
- 딥러닝
- mode collapse
- Generative Model
- deeplearning
- 디지털신호처리
- controllable GAN
- image
- Gan
- OS
- feature
- 운영체제
- Building Basic GAN
- depth
- DSP
- Depth estimation
- Deep learning
- 3d object detection
- ML
- TRACKING
- AI
- design pattern
- pcb
- Total
- Today
- Yesterday
일 | 월 | 화 | 수 | 목 | 금 | 토 |
---|---|---|---|---|---|---|
1 | 2 | 3 | 4 | 5 | ||
6 | 7 | 8 | 9 | 10 | 11 | 12 |
13 | 14 | 15 | 16 | 17 | 18 | 19 |
20 | 21 | 22 | 23 | 24 | 25 | 26 |
27 | 28 | 29 | 30 |