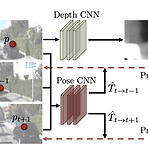
이 논문은 unsupervised한 방법으로 image depth를 구하는 모델을 학습시키는 방법이다. 학습에는 sequential한 이미지가 필요하고, 각 이미지의 pose를 이용해 한 이미지의 point들을 다른 이미지로 projection하고, projection된 위치의 점과 Intensity를 비교해 loss를 구한다. 여기서 pose도 model output이므로 gt없이 loss 계산이 가능해진다. Overview 조금 더 구체적으로 살펴보면 다음과 같은 단계로 나누어 생각해 볼 수 있다. 1. $t$에서의 이미지 $I_{t}$를 depth CNN에 통과시켜 Depthmap $\hat{D_{t}}(p)$를 구한다.(Depth map은 모든 이미지 픽셀에 대한 depth를 가지고 있다.) 2...
Deep Learning
2022. 2. 17. 00:22
최근에 올라온 글
TAG
- controllable GAN
- ML
- Generative Model
- design pattern
- depthmap
- depth
- 운영체제
- machine learning
- 디지털신호처리
- Building Basic GAN
- Operating System
- 3d object detection
- ML Pipeline
- DSP
- conditional GAN
- Depth estimation
- Gan
- TRACKING
- pcb
- Deep learning
- Raspberry Pi
- 딥러닝
- AI
- OS
- 신호처리
- image
- mode collapse
- MLOps
- deeplearning
- feature
- Total
- Today
- Yesterday
최근에 달린 댓글
링크
공지사항
일 | 월 | 화 | 수 | 목 | 금 | 토 |
---|---|---|---|---|---|---|
1 | 2 | 3 | 4 | |||
5 | 6 | 7 | 8 | 9 | 10 | 11 |
12 | 13 | 14 | 15 | 16 | 17 | 18 |
19 | 20 | 21 | 22 | 23 | 24 | 25 |
26 | 27 | 28 | 29 | 30 | 31 |
글 보관함