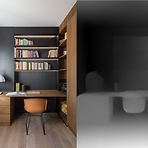
환경 설정 tflite설치가 가능한 가장 최신 버전이 python인데 os에는 3.9만 깔려 있어서 virtualenv에서 쓰기 위해 다른 버전을 설치해야 한다. 아주 귀찮게도 3.8버전은 distutils 이슈가 있고, 3.7버전은 apt-get으로 설치가 안된다. 따라서 아래 방법으로 직접 빌드해야한다. (repository추가해서 하고 싶었는데 linux repo를 함부로 넣지 말라는 글을 보고 포기했다. 에러가 나기도 했고..) sudo apt-get install -y build-essential tk-dev libncurses5-dev \ libncursesw5-dev libreadline6-dev libdb5.3-dev libgdbm-dev libsqlite3-dev \ libssl-dev ..
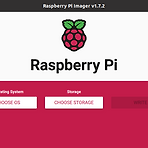
우분투를 사용하여 설치해서 우분투를 기준으로 기록한다 Raspberry Pi Imager를 사용하면 편하다. Raspberry Pi OS – Raspberry Pi From industries large and small, to the kitchen table tinkerer, to the classroom coder, we make computing accessible and affordable for everybody. www.raspberrypi.com 다운로드 받아서 설치하면 된다. 이미저를 실행시키면 아래와 같은 창이 뜬다. Operating system에서 raspberry pi o(32-bit)를 선택하고, storage에서 os를 설치할 sd card를 선택하면 된다. 터미널에 lsblk ..
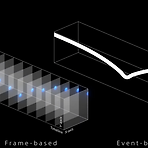
Event-based Camera란 이미지의 변화를 인식해 기록하는 카메라이다. 실제 인간의 눈은 빛의 변화를 더 잘 인식하도록 되어있는 것에서 아이디어를 착안해 만든 것이라고 한다. 이 카메라는 Intensity에 변화가 생기는 이미지 좌표와 시간을 저장한다. 따라서 변화가 많은 이미지일 수록 저장되는 데이터 양이 많아진다. 위에 이미지 처럼 공이 튀는 장면이라면, 공이 움직이는 위치와 시간이 저장되는 것이다. Frame-based 카메라와 비교했을 때, 모든 픽셀을 다 저장해야 하는 frame-based camera보다 변화만을 저장하는 event-based camera가 더 작은 크기를 가지고, 속도도 더 빠르다. 따라서 event-based camera는 high resolution, heigh ..
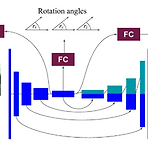
이 논문은 하나의 이미지의 depth, egomotion, object motion, and camera intrinsics을 동시에 학습하는 방식을 제안한다. motion-prediction network는 camera motion, motion of every pixel with respect to the background, and the camera intrinsics(focal lengths, offsets and distortion) 학습하고, 다른 network는 depth maps을 학습한다. 또한 occlusion 변화를 반영하기 위한 loss도 제안한다. 전체적인 Loss를 구하는 방식과 개념은 이전에 정리했던 논문에 기반하므로 이것을 참고하면 도움이 될 것이다. Learning the..
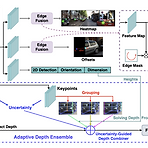
world center구하려면 3D object의 image에 투영된 center(uc, vc)와 world depth(z)가 필요하다. 이 논문에서는 크게 이 두 가지를 분리해서 구한다. 이 논문은 CenterNet의 확장이라고 볼 수 있다. CenterNet은 heatmap head, offset head, dimension head로 나누어져있다. heatmap head, offset head는 비슷한 기능을 하지만 3D point를 구하고, 이미지 안쪽과 이미지 바깥쪽에 있어 occlusion된 object를 구분하여 center를 구한다는 차이가 있다. Dimension head는 dimension orientation, 2d detection도 같이 구한다는 차이가 있다...
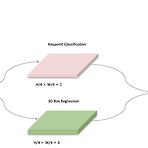
이 논문은 이미지에서 인식된 obeject(차량)의 실제 bounding box를 중심/크기/각도로 나누어 정보를 얻어 구할 수 있게 하는 모델을 제안하고 있다. 하나의 이미지에서 차량의 실제 정보를 얻을 수 있는(3D object detection) one-stage architecture를 제안하였으며, 중심/크기/각도로 분리하여 구하는 방식(multi-step disentanglement approach)을 제안하였다. Backbone - DLA(Deep Layer Aggregation)-34 수정해서 사용 - 모든 hierarchical aggregation을 DCN(Deformable Convolutional Networks)으로 대체 hierarchical aggregation은 같은 계층의 ..
수강 강의: 선형대수, 이상화 교수님 수강 기간: 2021.08 ~ 2021.11
Summary · Highly motivated graduate school applicant with particular interest in computer vision · Constantly study deep learning by reading relevant papers and implementing code · 2+ years of work experience in camera based Self-driving field Work Experiences Junior Algorithm Engineer - 02/2020 to present Stradvision, Seoul · Investigated methods to enhance accuracy of object’s features measure..
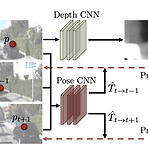
이 논문은 unsupervised한 방법으로 image depth를 구하는 모델을 학습시키는 방법이다. 학습에는 sequential한 이미지가 필요하고, 각 이미지의 pose를 이용해 한 이미지의 point들을 다른 이미지로 projection하고, projection된 위치의 점과 Intensity를 비교해 loss를 구한다. 여기서 pose도 model output이므로 gt없이 loss 계산이 가능해진다. Overview 조금 더 구체적으로 살펴보면 다음과 같은 단계로 나누어 생각해 볼 수 있다. 1. t에서의 이미지 It를 depth CNN에 통과시켜 Depthmap ^Dt(p)를 구한다.(Depth map은 모든 이미지 픽셀에 대한 depth를 가지고 있다.) 2...
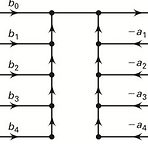
Filter라고 하는 어떤 system을 디자인하고 구현 할 때, filter structure를 통해 실제로 어떻게 구현할 것이며, 어떻게 디자인할 것인지를 고려해볼 수 있습니다. Basic Elements 이 포스팅에서는 LTI system을 고려하며, filter structure는 아래와 같은 요소들로 구성됩니다. Adder: 두 선이 만나는 점으로 표현된다. Multiplier(gain): 화살표 위에 적힌 값을 곱해준다. Delay element (shift or memory): 화살표 위에 z−k가 적혀있다면, k만큼 shift된 것이다. IIR Filter Structure IIR(Infinite-duration Impulse Response) filter는 입력신호의 값과 출..
- design pattern
- pcb
- Raspberry Pi
- MLOps
- controllable GAN
- Operating System
- Generative Model
- 신호처리
- feature
- ML
- depth
- mode collapse
- AI
- Building Basic GAN
- 디지털신호처리
- 운영체제
- Gan
- 딥러닝
- ML Pipeline
- DSP
- conditional GAN
- OS
- Depth estimation
- TRACKING
- Deep learning
- machine learning
- 3d object detection
- deeplearning
- image
- depthmap
- Total
- Today
- Yesterday
일 | 월 | 화 | 수 | 목 | 금 | 토 |
---|---|---|---|---|---|---|
1 | 2 | 3 | 4 | 5 | ||
6 | 7 | 8 | 9 | 10 | 11 | 12 |
13 | 14 | 15 | 16 | 17 | 18 | 19 |
20 | 21 | 22 | 23 | 24 | 25 | 26 |
27 | 28 | 29 | 30 |